Will Generative AI Suppliers Last Long Enough for Games to Ship? | AI and Games Newsletter
In this weeks newsletter, we address the elephant in the room.
Hello all, welcome to this weeks edition of the AI and Games newsletter. This week, we’re going to talk about the recent news regarding Stability AI, and how it highlights a fundamental problem for the adoption of generative AI in video games.
But before we get into that, let’s cover some announcements!
But First, a Word From Our Sponsor…
Special thanks to our sponsor of this weeks newsletter: modl.ai
modl’s ‘AI Engine’ provides AI-driven bots that can discover bugs, crashes, and performance issues. Plus your modl bots can embody player behaviour for more personalized game experiences.
The modl AI engine is available now for both Unity and Unreal game engines. With modl’s bots coming to your aid, game testing will never be the same again.
Try modl:test today by visiting https://modl.ai/sales-signup/
Weekly Announcements
Here’s everything else happening around AI and Games this week that you need to catch up on!
A big thank you to Elaine Dowman and the team at Barclay’s for having me down at their event last week to discuss AI for games alongside Rupam Dave from Harbottle and Lewis. It was well received, and I’m busy preparing for the next big event.
Speaking of, I’ll be hosting a roundtable on AI for games at the Barclay’s Game Frenzy on May. You can find out more about the event here.
No further speaking engagements planned until the Artificial Intelligence and Games Summer School in June and then Develop: Brighton in July at the moment. But, as always if you’re interested in my services (for speaking or otherwise), visit my Professional Services page.
Now without further ado, let’s get into this weeks main story…
Stability AI’s Woes Highlight the Risks of Generative AI for Games
This week’s deep dive focusses on the recent news stories surrounding Stability AI - the creators of image generator Stable Diffusion. The focus is not necessarily on the company themselves, but rather how their recent fortunes are emblematic of a larger problem surrounding a lot of AI companies at the moment. Perhaps more critically for this audience, we dig into how these issues are going to be a problem in game development.
Stability’s Financial Woes
UK-based Stability AI made headlines in the past couple of weeks courtesy of the deep-dive expose by Forbes. While the crux of the story was about Stability AI’s founder Emad Mostaque, the more interesting headline for me as with regards to the company finances.
You can get into the details via the Forbes article, or The Register if you prefer, but the real story for me was how the company spent close to $160 million only to bring in revenues of approximately $11 million. I’m no mathematician - I mean I’m a computer scientist, which makes me a lazy applied mathematician - but those numbers simply don’t stack up!
Digging a little deeper, you can see that this breaks down into two distinct areas:
Salary costs for all of Stability’s staff, totalling $54 million.
The cost of hosting, training and providing access to Stability’s AI models at $99 million.
Now I’m not going to decry anyone for getting that nice big pay packet - AI is a hot topic right now, you do you - but the costs of their AI models (largely from hosting them on Amazon Web Services) is as staggering as it is commonplace.
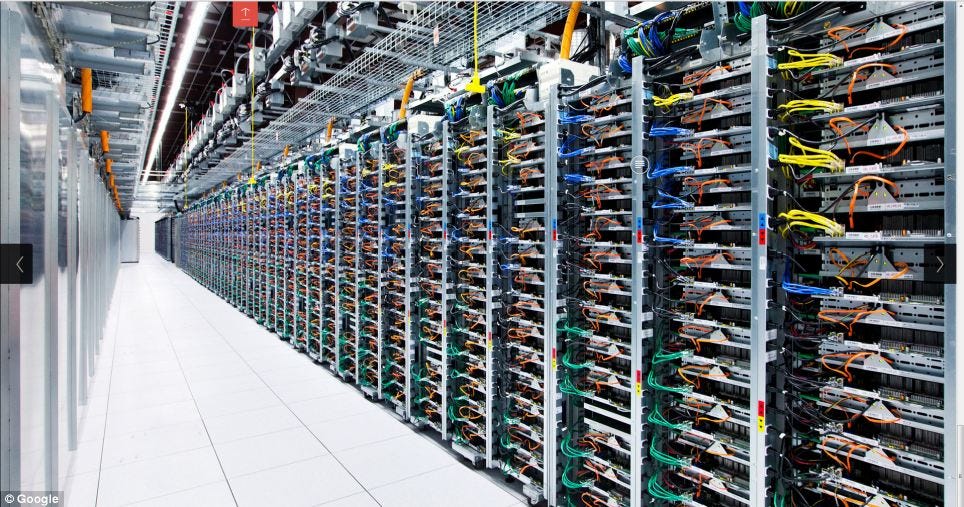
The (Financial) Cost of Generative AI
The cost of hosting for one year at Stability may be in part due to Mostaque’s rather unfocussed business strategy (see the Forbes article for more on that), but it is reflective of the market and the technology as a whole.
By the way for anyone keen to do the math, that $99 million equates to $270,000 a day for all of their work in building a variety of generative AI models. It’s worth stating that Stable Diffusion is not the only model that Stability AI develop, they have a variety of models for generating images, video, audio, 3D models, and a number of text generation systems (i.e. Large Language Models or LLMs) in their portfolio.
But it’s also not uncommon for these larger companies to be footing that kind of web hosting bill. In fact last year, estimates suggested that GPT creators OpenAI spend roughly $700,000 a day to host their language models (which going the other way is $255,500,00 a year).
Why are these numbers so astronomically large? I mean I’m throwing these numbers around like confetti, but at the end of the day that’s a lot of money for something whose quality could be justifiably described as ‘variable’. Naturally I’m not privy to the inner workings of these businesses, but it’s worth highlighting that hosting and training many generative models is prohibitively expensive due to the sheer size and scale of them. Given the number of parameters utilised in these models, they are typically hundreds of gigabytes in size, and require processing capability far beyond individual computers to run much less train in a practical timeframe. Hence the reliance on the Amazon Web Services in some instances, rather than building up their own cloud compute infrastructures.
Similarly, this requirement is why many 3rd party generative AI tools such as Jasper and Chibi.AI are effectively ‘wrappers’ for the likes of GPT and Stable Diffusion. Given it’s more cost effective to interface with these systems and try to get them to do that which your product advertises, than running your own models for that specific business use case.
And so yes, this is all very expensive. But what does it have to do with games? Well there’s three things to unpack here:
Stability AI is indicative of many a generative AI company that is unlikely to turn a profit any time soon. Many of which will require further investment to build a strong ROI and survive the coming years.
With increasing regulation on the horizon, be it on platforms or at national and international level, generative AI companies will need adapt to survive.
Lastly, video games are a long-term financial proposition, and are therefore incredibly risk-averse as they attempt to weather dynamic and volatile tech markets.
And so the question is, how can generative AI truly make an impact in the games industry if the companies pioneering the sector are increasingly unlikely to survive the duration of a AAA project’s development?
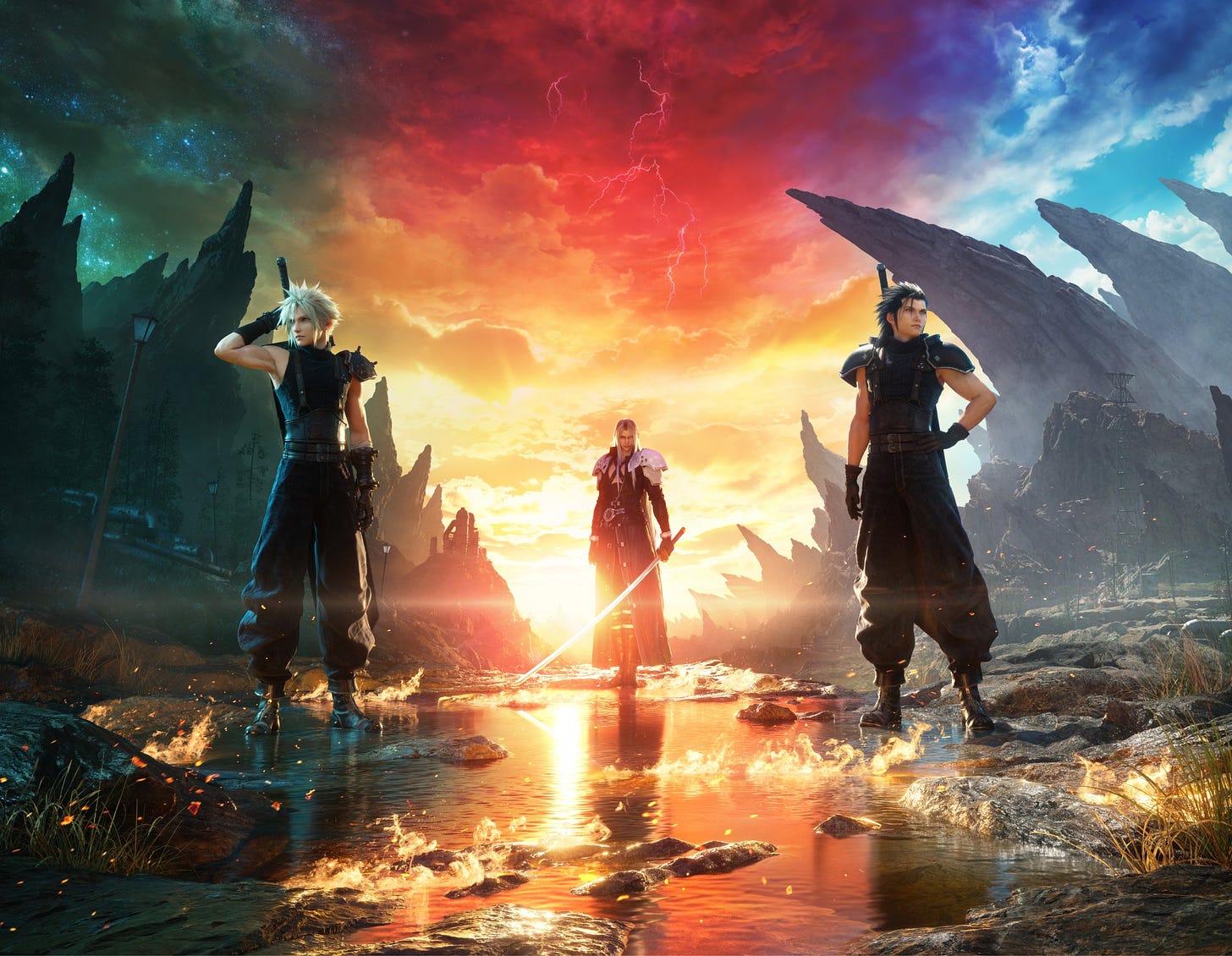
The Risks of Generative AI for Games
As always, I come across as a cynic when it comes to generative AI for games, when the truth is I’m more of a cautious optimist. I see the potential this technology has in the future, but right now there is simply too much risk for many game developers to consider adopting it within their pipeline.
It’s worth stressing before I dig into this further, that it does not mean that companies are not exploring it in detail. Just last week I discussed Ubisoft’s NEO NPC, which relies on conversational AI powered by Inworld. Plus at GDC 2024 we had companies such as Keywords Studios talk about their work to build entire games using only generative AI tools. This particular example, Project AVA, is notable in that the studio successfully built a relatively small albeit complete game in 2023. Despite this achievement, they have zero intent to release it at this current time given the legal and ethical issues it raises. Outside of this, every AAA developer I talk to is experimenting with their own tools and systems derived from generative AI technologies. But the risks involved are simply too much to consider use in production at scale.
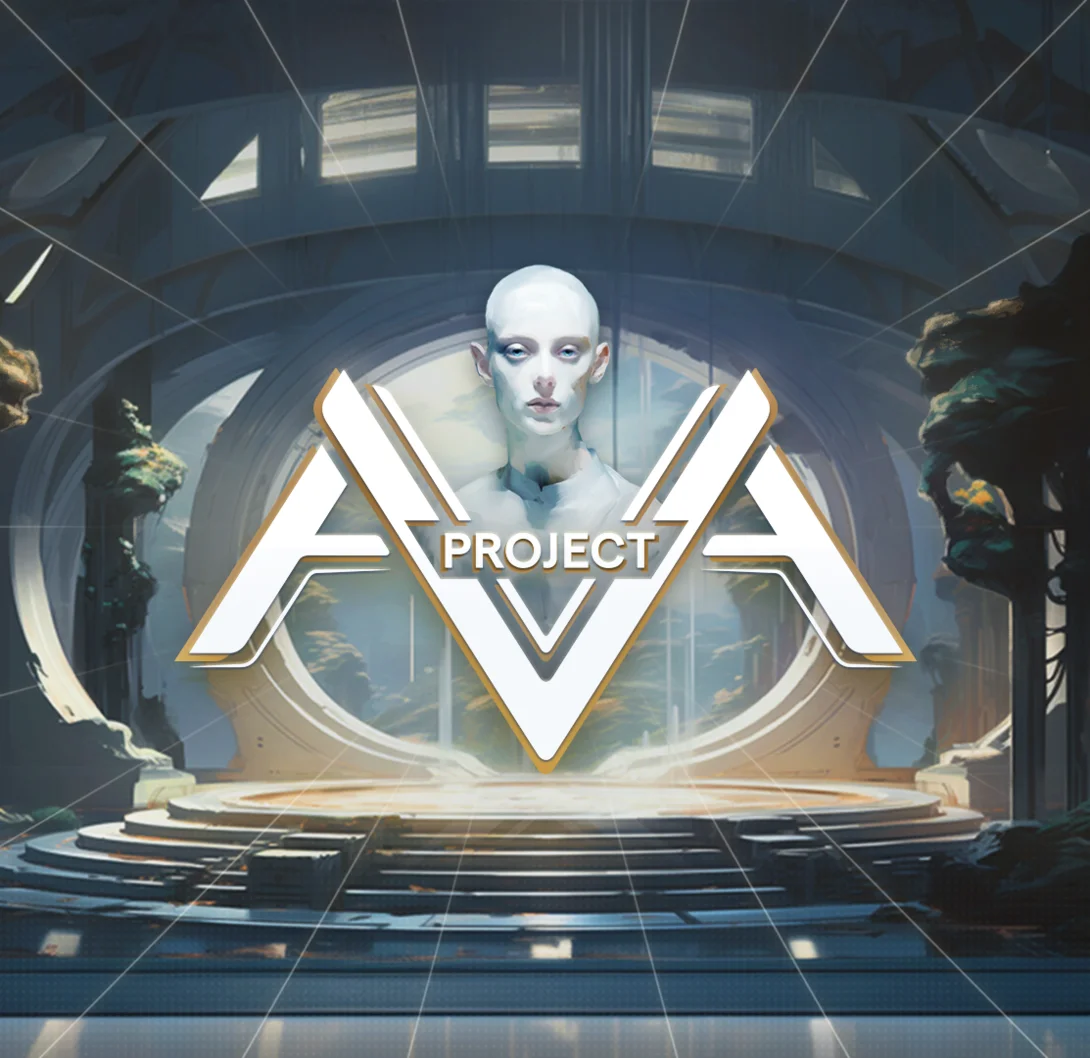
Risk is a very ugly word to throw around in video games, because the industry is incredibly risk averse. While we see a myriad of diverse and exciting games emerge across the sector, with creatively rich titles such as Balatro breaking 1 million sales, and Viewfinder and Venba receiving awards at last weeks BAFTA Games event, the majority of the industry is very mindful of the types of games it is making (or funding), and the costs it incurs.
If we consider a list of what are the biggest (critical and commercial) AAA games of 2024: Final Fantasy VII Rebirth, Dragon’s Dogma 2, Tekken 8, Like A Dragon: Infinite Wealth, Persona 3 Reload and Helldivers 2. They all have several traits in common:
They’re all either sequels or remakes of existing IP
For context: Final Fantasy VII Rebirth is the 2nd entry of a planned trilogy of remakes of the 1997 game Final Fantasy VII. Personal 3 Reload is a ground-up remake of Persona 3 from 2006.
Like A Dragon: Infinite Wealth is confusingly the 9th mainline entry of the Yakuza series. The franchise is now embracing the translation of its Japanese name (Ryū ga Gotoku) for more recent entries.
They are all taking established concepts and ideas from the industry and reworking them to modern sensibilities and audiences.
They all had extensive multi-year development lifecycles.
Helldivers 2 was confirmed to have taken just shy of 8 years to build.
While Final Fantasy VII Rebirth came to market 4 years after its predecessor - Final Fantasy VII Remake — it was in development as early as 2019.
It’s this latter point that underpins the risks of generative AI when it is provided by outside vendors. There are a whole bunch of issues I don’t want to get into today given I simply don’t have time - notably protection of intellectual property rights, ownership and copyright of generated assets, reliability of generated output in context of platforms and age ratings, plus of course the legal issues of generative AI providers and the ethical issues packed in with that. Today my focus is on something highly games specific: technical debt and production timelines.
Minimising Risk in Long Production Timelines
As games move into full production, there is a huge emphasis on minimising risk in all facets of the pipeline. Critically, this includes all of the technical components. This can include the primary game engine being adopted (be it in-house or 3rd party), the proposed platform(s) for launch (given they influenced overall technical budgets), the middleware and tools utilised for development, and much more.
A lot of generative AI adoption I see when working with studios tends to fall into early ideation/iteration phases. Meaning that it’s designed to help speed up that early phase of development. It’s not used when the team gets into the meat of production, and there’s a number of issues that lead to this conclusion:
Tools don’t fit needs of the development process/pipeline.
And critically, they’re not good enough to merit a change to an established process.
The tools are often provided without an enterprise license, meaning studios repeatedly have to bill for monthly subscriptions and don’t ‘own’ their copy of the tool outright.
Critically, most generative AI tools are hosted on 3rd party web platforms. Meaning the studio is reluctant for two reasons:
Their assets are being submitted to a 3rd party who perhaps cannot be trusted with their intellectual property.
In the event that the 3rd party goes out of business, the tool is no longer available.
Stories such as Stability AI having financial troubles only help reinforce this concern. Games studios need partners to be in business for years to help build their own internal expertise of those tools/systems and rely on their external support. Then in the event things go south, the tool could still be used without the support of the original provider if that experience is baked into the team.
Perhaps one of the best examples of this in recent years is the release of Helldivers 2 (released in February of 2024) and 2022’s Warhammer 40K: Dark Tide. Both games, were developed and brought to market using the Autodesk Stingray game engine. This is particularly notable, given Autodesk discontinued support for the engine in 2018.
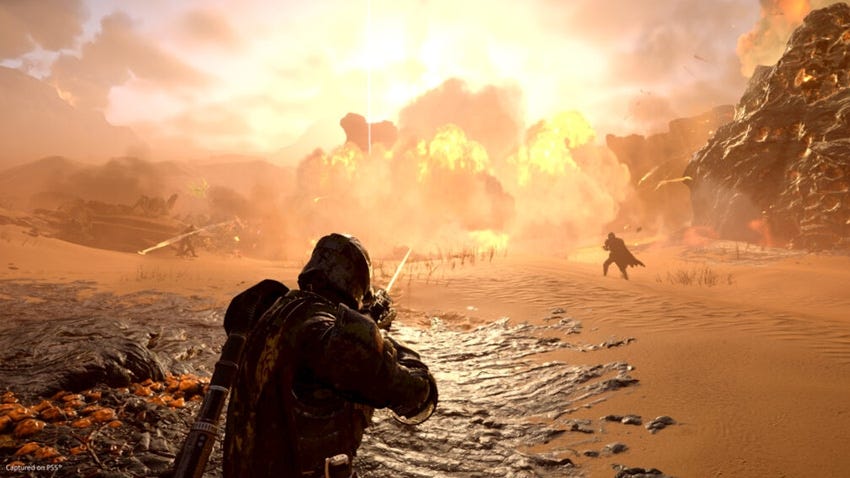
Now this might sound risky, but it fits with their own internal skillsets and pipeline.
Both studios (Arrowhead Game Studios and Fatshark) have released multiple games using the engine - meaning they know it well and are willing to commit to it despite its discontinuation.
The engine source was available to them to modify and extend in ways that made sense to them. Rather than them being locked out by of the tool by a 3rd party provider.
On that note: it’s worth raising that Stingray was originally called BitSquid, with the company being owned by the founders of Fatshark. So that studio has invested in that engine since the beginning.
Compare this to an external generative AI tool that is accessible only via a web-API (or similar front page). Once those servers go down, the tool is no longer accessible. If a studio cannot see the viability of your tool in the worst case scenario of you going bust, then their willingness to adopt it will reduce drastically.
The Generation of AI Stability
So what does all of this mean in the grand scheme of things? Critically, we need to see more generative AI providers show not only that they have a stable business model and are capable of growth, but that they are capable of providing support for studios in ways that better align with their production risks. Can they provide full source code access? Can they offer better offline tooling? Anything that allows for studios to see a viable way to continue to use their offerings in the event things go horribly wrong for the vendor.
Stability AI’s recent financial difficulties highlight that its rise to prominence and then potential collapse could have occurred in less time than it took to make Final Fantasy VII Rebirth. If a tool provider cannot convince a studio that they’re going to survive their production timelines, what chance do they have of being adopted?
Wrapping Up
That’s us for this week! Right now there’s plenty of AI stories to dig into that are on the horizon, be it Google’s SIMA project, the recent update to Meta’s Llama language model, but we’ve got some classic game AI content coming up on AI and Games, and fingers crossed we can start sharing it next week. See you then!
This analysis of Generative AI is truly intriguing. While most focus on technological advancements, this one takes a unique business perspective in examining the feasibility of current implementation of such technology. I believe the variables analyzed, such as the degree of reliability with the provider, the feasibility of implementing a certain tool in a process, and its potential benefits, are key aspects that allow us to keep our feet on the ground and better evaluate whether all these technological advances can truly help us improve workflows in different contexts.